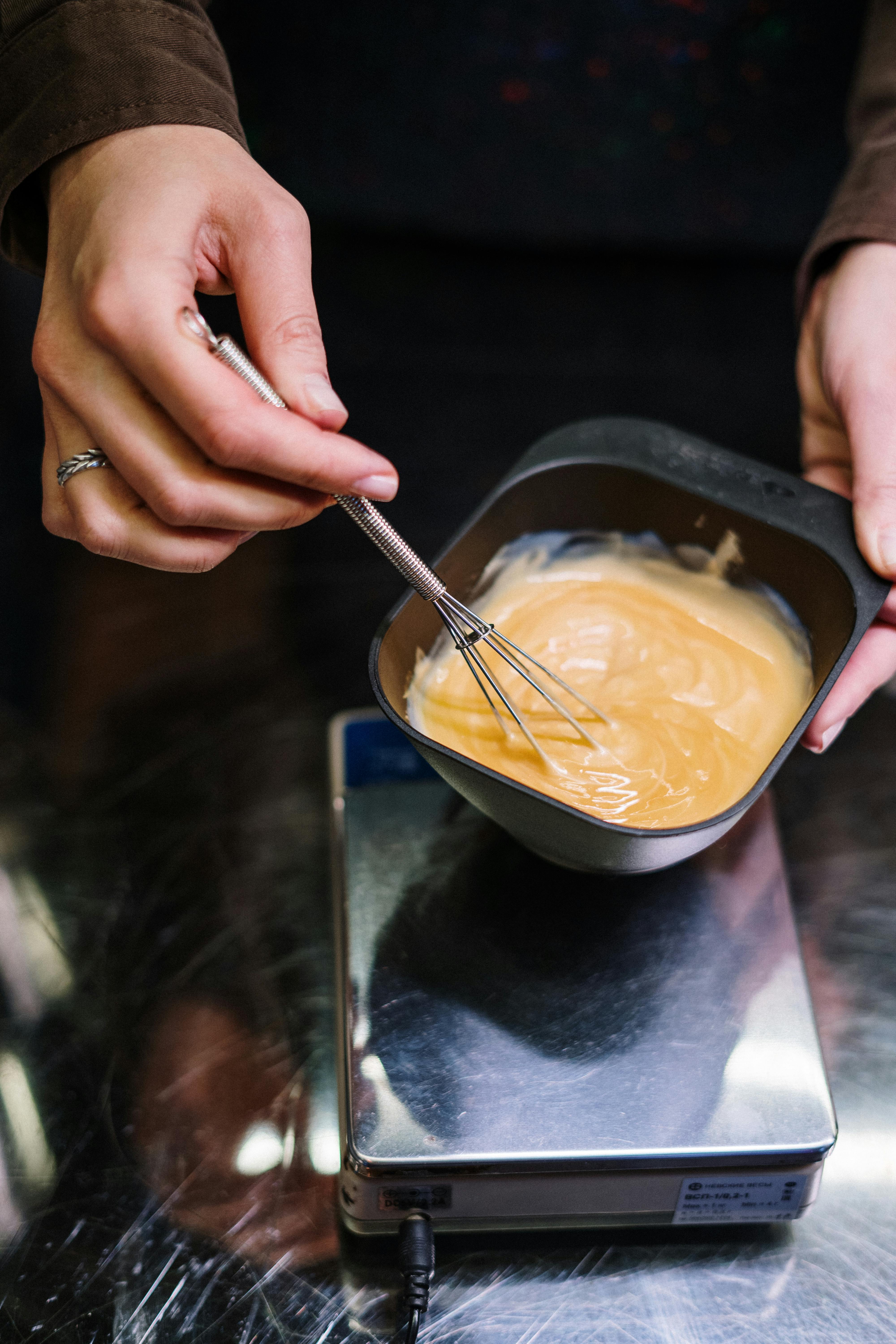
How to Calculate Degrees of Freedom in Statistics
Understanding Degrees of Freedom
In statistics, the concept of **degrees of freedom** plays a crucial role in various analyses, such as hypothesis testing or estimating parameters. It is particularly important when distributing values within a given sample data set. Degrees of freedom (df) generally represents the number of independent values that can vary in an analysis without breaking any constraints. **Calculating degrees of freedom** accurately is vital for ensuring the validity of statistical tests. Mastering this concept helps in grasping not just the foundational statistics but also the advanced methods and applications in research.
What is Degrees of Freedom?
The **definition of degrees of freedom** is often foundational. In simple terms, it quantifies the amount of independent information available in a dataset for estimating statistical parameters. For instance, in a sample of size \( n \), the degrees of freedom for a single mean calculation would be \( n-1 \). This adjustment accounts for the fact that one free parameter (the mean) has already been estimated from the data. Understanding **degrees of freedom explained** is crucial for statisticians and researchers, as it impacts both the **significance** of tests and the interpretation of results.
Importance of Degrees of Freedom
The **importance of degrees of freedom** cannot be overstated. They are significant in statistical tests, such as the t-test or ANOVA (Analysis of Variance). In these contexts, the degrees of freedom determine how the critical values are identified from their respective distributions. A higher degree of freedom indicates less variability in the parameter estimates, thus enhancing the reliability of statistical inference. The **impact of degrees of freedom on statistical tests** can make a substantial difference in decisions made based on data analyses.
Degrees of Freedom and Sample Size
The relationship between **degrees of freedom and sample size** is straightforward: generally, the larger the sample size, the greater the degrees of freedom available. This is important when considering the robustness of the statistical tests being used. In studies using smaller samples, the restrictions imposed on degrees of freedom can lead to skewed results, diminishing the test's power. Understanding how both elements interact can significantly impact the design of experiments and resulting conclusions.
Calculating Degrees of Freedom for Different Tests
Different statistical tests require specific methods for **calculating degrees of freedom**. Understanding these calculations is essential for correctly applying statistical tests and for accurate analysis of results. Here we discuss some common scenarios and how to derive the degrees of freedom in each case, including t-tests and ANOVA.
Degrees of Freedom for T-Test
<pThe **degrees of freedom for t-test** calculations depend on the type of t-test being used. For an independent samples t-test, the formula used is \( df = n_1 + n_2 - 2 \), where \( n_1 \) and \( n_2 \) are the sizes of the two samples being compared. This is essential because it helps to determine the t-distribution used for significance testing. **Understanding degrees of freedom in t-tests** is vital for accurate interpretation of statistical results and findings.Degrees of Freedom for ANOVA
In ANOVA, the **degrees of freedom for ANOVA** are calculated based on the number of groups and sample sizes. It requires two components: between-group degrees of freedom (\( df_{between} = k - 1 \), where \( k \) is the number of groups) and within-group degrees of freedom (\( df_{within} = N - k \), where \( N \) is the total number of observations). This structure is crucial as it lays the groundwork for the F-statistic calculation, further demonstrating the **degrees of freedom significance** in data analysis across multiple sample groups.
Degrees of Freedom in Chi-Square Test
In a **chi-square test**, the degrees of freedom are determined differently based on the test type: for goodness of fit, \( df = k - 1 \) (where \( k \) is the number of categories), while for a contingency table, \( df = (r - 1)(c - 1) \) (with \( r \) being the number of rows and \( c \) the number of columns). **Calculating degrees of freedom in chi-square tests** is pivotal in interpreting categorical data effectively.
Practical Examples of Degrees of Freedom Calculations
Explaining the concept of degrees of freedom often necessitates practical examples. Let’s explore a few scenarios that showcase how to **calculate degrees of freedom** in various statistical contexts.
Example 1: Single Sample Mean
Consider a situation where a sample of 10 test scores is taken. The calculation for the **degrees of freedom calculation** for this setting would follow the formula \( df = n - 1 \). Therefore, the degree of freedom would be \( 10 - 1 = 9 \). This example illustrates how simply deriving degrees of freedom offers insights into the variability of this sample mean in wider applications.
Example 2: Two-Sample T-Test
Suppose we have two independent samples, with sizes 8 and 12. Utilizing the previously discussed formula, we find \( df = 8 + 12 - 2 = 18 \). This means our t-tests will refer to a critical t-distribution with a degrees of freedom of 18, helping in evaluating significance levels appropriately. This **practical example of degrees of freedom** demonstrates the method's application in real situations.
Example 3: ANOVA with Three Groups
In an ANOVA test involving three treatment groups with a total of 30 observations, we calculate the degrees of freedom as \( df_{between} = 3 - 1 = 2 \) and \( df_{within} = 30 - 3 = 27 \). This shows how these two figures interact in understanding the variances, highlighting the importance of accurate df calculations in **statistical methods degrees of freedom** applications.
Key Takeaways
- Degrees of freedom count the number of values that are free to vary in a statistical analysis.
- They play a crucial role in controlling for potential biases in various statistical tests.
- Calculating degrees of freedom varies from one statistical test to another, from t-tests to ANOVA and chi-square tests.
- Practical examples can greatly enhance understanding of this concept in real-world scenarios.
- Accurate degrees of freedom calculations are essential for valid statistical inferences.
FAQ
1. How do you calculate degrees of freedom in regression analysis?
In regression analysis, calculating **degrees of freedom in regression** typically involves determining \( df_{model} = k - 1 \) and \( df_{error} = N - k \), where \( k \) is the number of predictors and \( N \) is the total number of observations. This helps in evaluating the regression model's performance.
2. What is the relationship between degrees of freedom and the chi-square distribution?
The **relationship between degrees of freedom and the chi-square distribution** is fundamental in conducting chi-square tests. The chi-square statistic approaches a normal distribution as the degrees of freedom increase, enabling the interpretation of results with larger sample sizes.
3. Why are degrees of freedom important in hypothesis testing?
Degrees of freedom are crucial in **hypothesis testing** because they affect the critical values or cutoffs needed to determine significance. They dictate the shape of the distribution, impacting the probability of committing Type I and Type II errors.
4. Can degrees of freedom be adjusted?
Yes, **degrees of freedom adjustments** can be necessary in specific contexts, such as when dealing with finite sample corrections or when applying certain methods like complex data structures. Adjusting df appropriately ensures the calculations remain reliable and applicable.
5. How does sample size relate to degrees of freedom in data analysis?
The **impact of sample size on degrees of freedom** is significant; larger samples generally lead to higher degrees of freedom, reducing estimate variability in statistical analysis. However, small samples may introduce biases, necessitating careful interpretation of df within analyses.