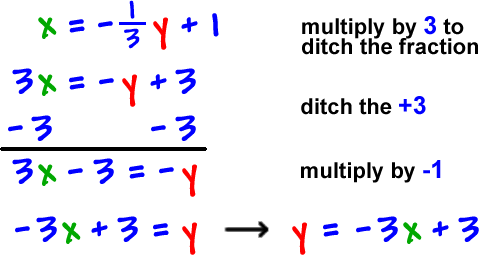
Apply Now
Effective Methods for Solving Differential Equations in 2025
Differential equations play a pivotal role in mathematics and various applications in science and engineering. In 2025, an array of sophisticated techniques has emerged to address the challenges of solving these equations effectively, including ordinary and partial differential equations. With advancements in computational tools and numerical methods, understanding how to approach these problems is essential for students and professionals alike. In this article, we will explore effective ways to solve differential equations, provide insights into their applications, and highlight some of the most promising methods available today. One of the key advantages of mastering differential equations is their extensive applicability across various fields such as physics, engineering, and even mathematical biology. By delving into different solution techniques, we can discover both analytical and numerical methods that cater to a wide spectrum of problems. Let’s explore a roadmap for this discussion that includes the categories of differential equations, effective solution methodologies, applications in real-world scenarios, and an outlook on the use of technology to enhance the solving process.Understanding the Types of Differential Equations
In order to effectively tackle differential equations, it's crucial to differentiate between the two main types: ordinary differential equations (ODEs) and partial differential equations (PDEs). An ODE involves functions of a single variable, while a PDE involves multiple variables. A thorough understanding of these distinctions is foundational for applying the correct solution techniques.Ordinary Differential Equations: A Closer Look
Ordinary differential equations are equations containing one or more derivatives of a function with respect to one independent variable. They are further categorized into linear and nonlinear equations. A linear differential equation takes the form: \[a_n \frac{d^n y}{dx^n} + a_{n-1} \frac{d^{n-1} y}{dx^{n-1}} + ... + a_1 \frac{dy}{dx} + a_0 y = f(x)\] Nonlinear equations, on the other hand, may involve terms that are products or powers of the dependent variable and its derivatives. Common methods of solving ODEs include separation of variables and the integrating factor method. Taking an example, consider the rate of change of population in a closed system modeled by the logistic equation: \[\frac{dP}{dt} = rP \left(1 - \frac{P}{K}\right)\] Setting up and solving such equations is critical for understanding systems in various fields including biology and economics.Partial Differential Equations: Key Insights
Partial differential equations (PDEs) describe phenomena involving functions of multiple variables. They present unique challenges in terms of boundary and initial value problems. For instance, Laplace's equation and the heat equation are classic examples of PDEs encountered frequently in physics. To solve PDEs, one often employs methods like Fourier series, separation of variables, or numerical techniques such as finite differences. For instance, the heat equation can be solved using Fourier transform methods, transforming a PDE into an ordinary differential equation, which is typically easier to manage.Boundary and Initial Value Problems
Both boundary value problems (BVPs) and initial value problems (IVPs) are critical for solving differential equations, especially in practical applications. BVPs involve finding a function that satisfies the conditions at two or more points, while IVPs specify initial conditions at a single point. A common example is the classic Sturm-Liouville problem where the solution needs to satisfy certain boundary conditions. The uniqueness of solutions in these contexts is secured by theorems that guarantee existence, thus ensuring stability in modeling real-world situations. The effectiveness of various numeric methods, including Runge-Kutta and Adams-Bashforth methods, is paramount in dealing with IVPs in complex systems.Analytical Techniques for Solving Differential Equations
When tackling differential equations, analytical methods often provide exact solutions and deeper insights into the structure of the models involved. Techniques like the Laplace transform and the Frobenius method for series solutions can effectively deal with a variety of differential equations.Using the Laplace Transform
The Laplace transform method is especially useful when converting differential equations to algebraic equations. This technique is applied widely in engineering to analyze linear time-invariant systems. The transformation simplifies the process of solving differential equations with time-dependent variables. For instance, consider the linear ODE: \[\frac{dy}{dt} + ay = b\] Applying the Laplace transform yields: \[sY(s) + aY(s) = \frac{b}{s}\] From here, we can solve for Y(s) and eventually use the inverse transform to find y(t).Separation of Variables Technique
The separation of variables technique can be employed to solve both ODEs and PDEs by isolating variable-dependent components. This technique is particularly effective for equations that can be rewritten in a form where each side is a function of a single variable. For example, consider a simple heat equation model that needs to describe temperature distribution over time. By separating the spatial and temporal variables, solutions can be derived by solving simpler ordinary differential equations.Numerical Methods for Solving Differential Equations
In many real-world applications, finding analytical solutions may not be feasible. In such cases, numerical methods become invaluable. These techniques allow for approximating solutions where traditional methods may fall short.Employing Runge-Kutta Methods
Runge-Kutta methods stand out as one of the most popular approaches for solving initial value problems. These methods iterate to produce increasingly accurate approximations of solutions. The fourth-order Runge-Kutta method (RK4) is particularly favored for its balance between complexity and accuracy. For instance, applying RK4 to the simple differential equation: \[\frac{dy}{dt} = f(t, y)\] involves computing several intermediate slopes to produce an accurate estimate of y at subsequent time steps.Euler's Method: A Simple Yet Powerful Tool
Euler’s method is a straightforward numerical technique used to solve ODEs. This method approximates the solution by evaluating the derivative at known points, allowing one to extrapolate to future points. While easy to implement, Euler's method can suffer from stability issues and should be used with caution, particularly in systems sensitive to initial conditions. For example, the application of Euler's method requires iteration over a small time step: \[y_{n+1} = y_n + hf(t_n, y_n)\] This approach demonstrates the trade-offs between simplicity and precision, especially in scenarios where high accuracy is essential.Applications of Differential Equations in Real Life
The applications of differential equations are vast and varied, impacting everything from engineering to biology. They help model physical systems, analyze behaviors in economies, and even predict biological patterns.Engineering and Physics Applications
In engineering, differential equations are critical in system dynamics to model vibrations and waves. The wave equation illustrates how differential equations describe sound, light, and mechanical waves. Addressing boundary conditions leads to real-world applications such as soundproofing and material strength predictions. H2 style provides insight into system behavior, revealing stability criteria and response characteristics, paving the way for innovations in control theory and optimization problems.Mathematical Biology: Modeling Ecosystems
Differential equations facilitate the modeling of population dynamics and interactions within ecosystems. Models like the Lotka-Volterra equations use differential equations to describe predator-prey interactions, thereby informing conservation strategies and ecological interventions. The exploration into chaos theory through differential equations reveals how small changes in initial conditions can lead to vastly different outcomes, a crucial concept in both ecological modeling and systems theory.Conclusion and Future of Differential Equations
As we continue to unravel the complexities of differential equations through innovative methodologies and computational advancements, the potential for deeper understanding is vast. The integration of machine learning and natural language processing into mathematical education serves as a significant leap forward, enhancing how we teach and apply these crucial concepts. By nurturing a strong foundation in techniques and applications, we prepare ourselves to leverage differential equations effectively in solving real-world problems. As this field continues to evolve, staying informed about emerging trends and technologies will be paramount.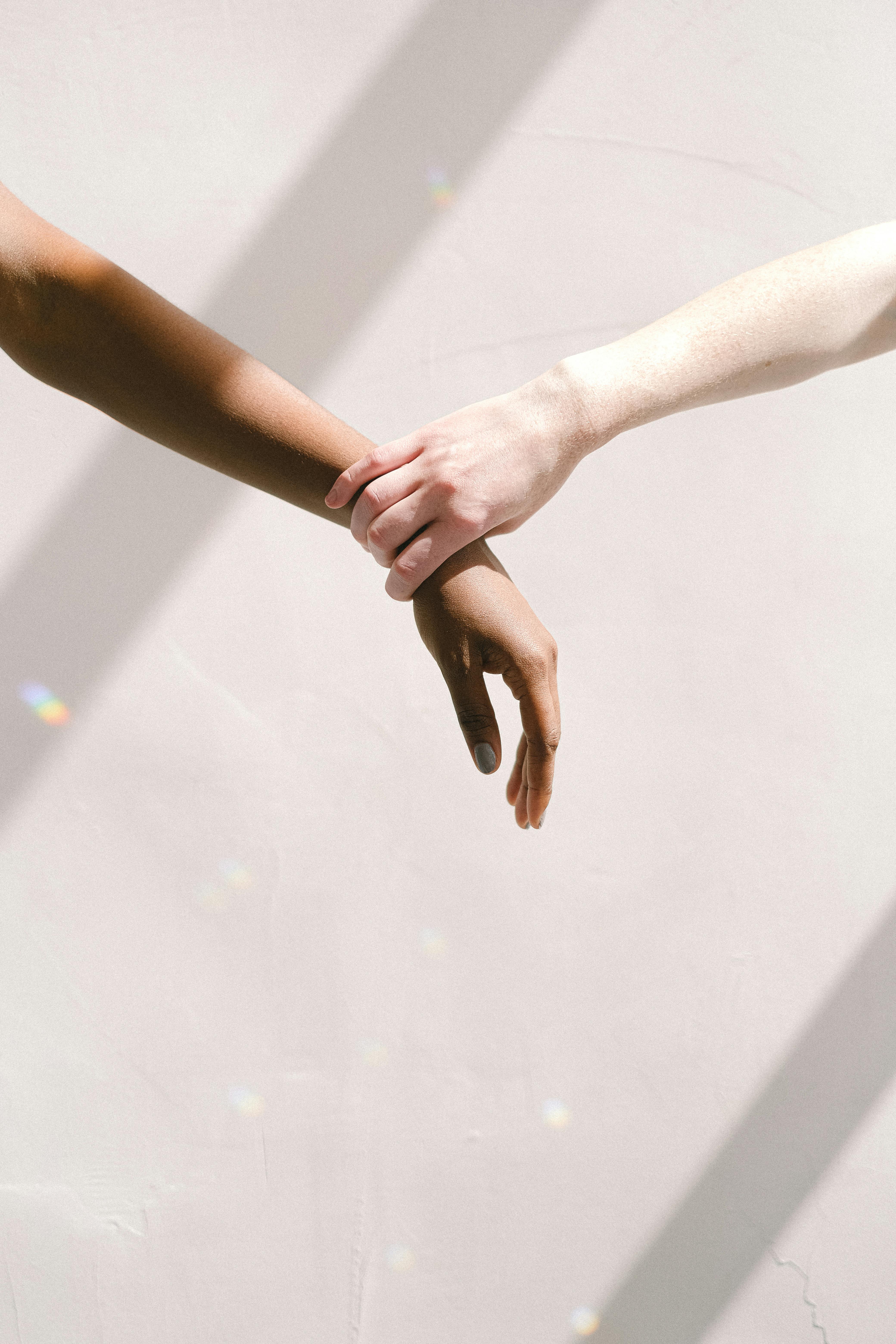
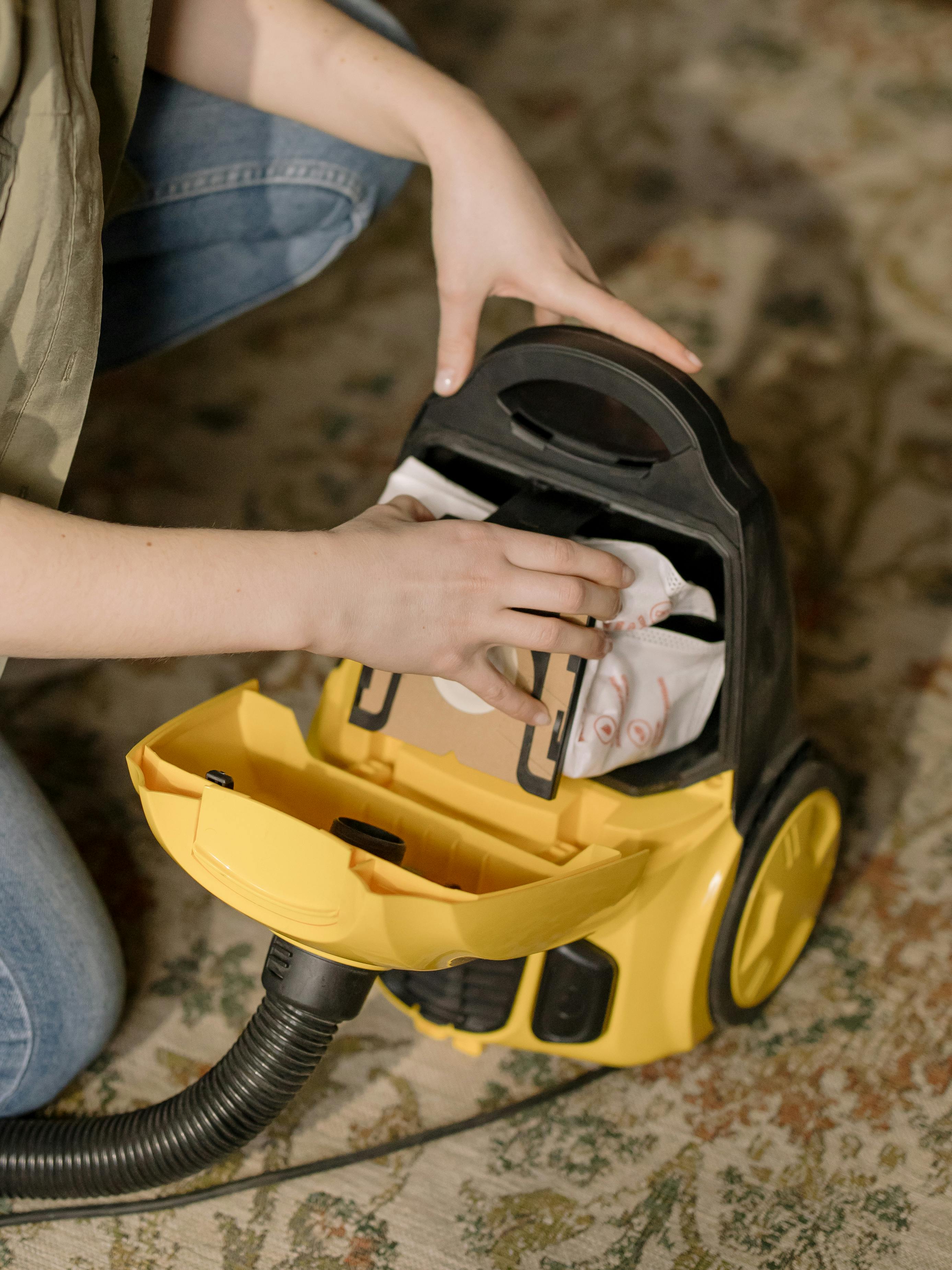