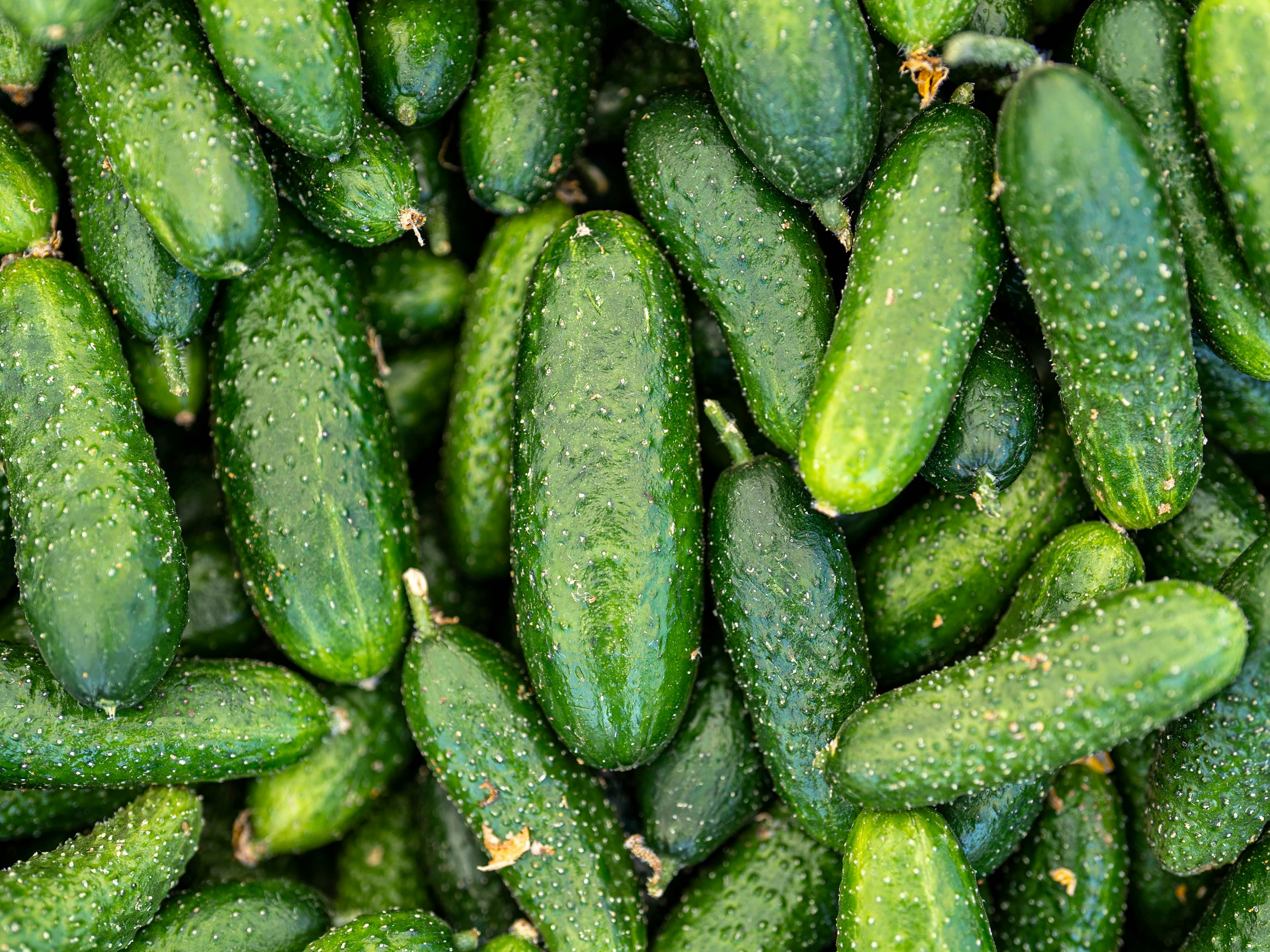
Effective Ways to Calculate Relative Frequency: A Practical Guide for Success in 2025

Understanding Relative Frequency in Data Analysis
Relative frequency is a fundamental concept in statistics, pivotal for interpreting data. It offers insights into the occurrence of events relative to the total number of observations. In practical terms, it provides a way to express the likelihood of specific outcomes, making it crucial for researchers and statisticians alike. By calculating relative frequency, one can understand patterns within the data and draw significant conclusions.
The importance of relative frequency extends into various fields such as business, healthcare, and social sciences. It allows professionals to make informed decisions based on empirical data rather than mere assumptions. Through the calculation of relative frequency, one can derive meaningful statistics and enhance data interpretation, supporting both descriptive and inferential statistical approaches. This article explores effective methods to calculate relative frequency, ensuring a strong grasp of this essential statistical technique.
We will explore the relative frequency formula, practical examples, and the importance of frequency tables in conducting accurate data analysis. Moreover, readers will find tips on avoiding common mistakes while calculating frequency ratios and an in-depth look into statistical significance and probability theory.
Key Methods to Calculate Relative Frequency
Calculating relative frequency involves a systematic approach that consists of several key methods. At its core, it requires determining the frequency count of an event and dividing it by the total number of observations. This straightforward calculation yields the relative frequency, a vital element of statistical frequency.
This process not only aids in understanding the dataset but also significantly improves frequency analysis. Here, we outline essential techniques and formulas that can be employed in calculating relative frequency.
1. The Relative Frequency Formula Explained
The relative frequency formula is expressed as:
Relative Frequency = (Frequency Count of Specific Event) / (Total Number of Observations)
This formula provides a simple and effective way to calculate relative frequency, allowing for quick analysis of statistical data. By using this formula, one can assess the likelihood of specific data outcomes, thereby facilitating informed decision-making.
When utilizing the relative frequency formula, it's crucial to ensure the total number of observations is accurate. Any discrepancies can lead to incorrect calculations, ultimately skewing the data interpretation.
2. Practical Examples of Relative Frequency Calculation
To illustrate how to calculate relative frequency, consider a scenario where a researcher surveys 200 individuals about their preferred modes of transportation. Let's say 80 individuals preferred driving, 50 preferred public transport, and 70 preferred biking. The calculations would be as follows:
- Relative frequency for driving: 80/200 = 0.4 or 40%
- Relative frequency for public transport: 50/200 = 0.25 or 25%
- Relative frequency for biking: 70/200 = 0.35 or 35%
These calculations provide a clear picture of each group's preference distribution, highlighting trends in transportation choices.
3. Utilizing Frequency Tables for Data Interpretation
Frequency tables are instrumental in displaying data in an organized manner, making it easier to calculate relative frequency. They present the frequency counts in relation to specific categories, enhancing clarity and enabling readers to grasp statistical frequencies effortlessly.
For effective usage of frequency tables, one can set up a table with categories on one side and their corresponding frequency counts on the other. Once established, calculating relative frequencies from each count becomes a straightforward task.
Here's a simple example of a frequency table for different sports preferences among 100 individuals:
Sport | Frequency Count | Relative Frequency (%) |
---|---|---|
Soccer | 40 | 40% |
Basketball | 30 | 30% |
Tennis | 30 | 30% |
This frequency table visualizes the relative frequencies, making it an effective tool for data representation in quantitative analysis.
Common Mistakes in Frequency Calculation
While the methods for calculating relative frequency appear straightforward, several common mistakes can lead to errors in analysis. Understanding these pitfalls is crucial to ensuring accurate statistical frequency counts and valid conclusions.
The most frequent mistakes include miscalculating total observations and failing to account for outliers. Such errors can obscure data interpretation or create a misleading representation of empirical frequencies. Additionally, not updating frequency counts after collecting new data can result in outdated analysis.
1. Overlooking Data Collection Techniques
Accurate frequency analysis begins with quality data collection techniques. Whether it's through surveys, experiments, or observational studies, researchers must ensure that data collected is inclusive, diverse, and relevant. Overlooking data collection methods can skew frequency ratios and lead to erroneous conclusions.
2. Ignoring Statistical Significance
Another critical mistake is neglecting the statistical significance of the calculated relative frequencies. Without analyzing whether the observed frequencies can be attributed to chance, researchers risk drawing unsubstantiated conclusions. Engaging in statistical tests to assess significance is vital for obtaining credible results in frequency analysis.
3. Misinterpretation of Data Results
Interpreting results inaccurately can lead to misinformed decisions and strategies. Thus, it's imperative to understand what relative frequency entails clearly. Familiarizing oneself with descriptive and inferential statistics ensures that data results are interpreted correctly, guiding effective data reporting.
Importance of Statistical Techniques in Frequency Analysis
Statistical techniques play a significant role in effective frequency analysis. They not only facilitate accurate calculations but also enhance data visualization and interpretation, making them crucial in research methods.
Advanced statistics, including techniques like probability distributions and sampling methods, provide a comprehensive understanding of frequency and empirical data. Utilizing these statistical analysis tools can significantly improve the reliability and validity of results.
1. Employing Data Analysis Methods
Data analysis methods such as descriptive and inferential statistics are vital in understanding frequency distributions. Descriptive statistics summarize data in a way that highlights patterns, while inferential statistics allow for generalizations to the broader population, improving frequency calculation accuracy.
2. Using Visualization Techniques
Data visualization techniques enhance the clarity of frequency distribution by showcasing the data in graphical formats. Charts, graphs, and histograms can vividly depict frequency counts and distributions, aiding in recognizing trends and making informed decisions.
3. Integrating Research Methods
The integration of various research methods enriches the understanding of frequency in statistics. Employing qualitative data analysis alongside quantitative methods allows for a holistic view of the data, ensuring accurate frequency measurement and analysis.
Final Thoughts on Calculating Relative Frequency
As we approach 2025, mastering the calculation of relative frequency is essential for success in data analysis. Through understanding key methods, avoiding common mistakes, and employing statistical techniques, researchers can significantly enhance their empirical frequency measurements.
In conclusion, whether you are working on qualitative data analysis or advanced statistical techniques, a firm grasp of relative frequency calculation will prove invaluable. With practical applications found in various fields, confident competency in frequency analysis will elevate your analytical abilities to new heights.
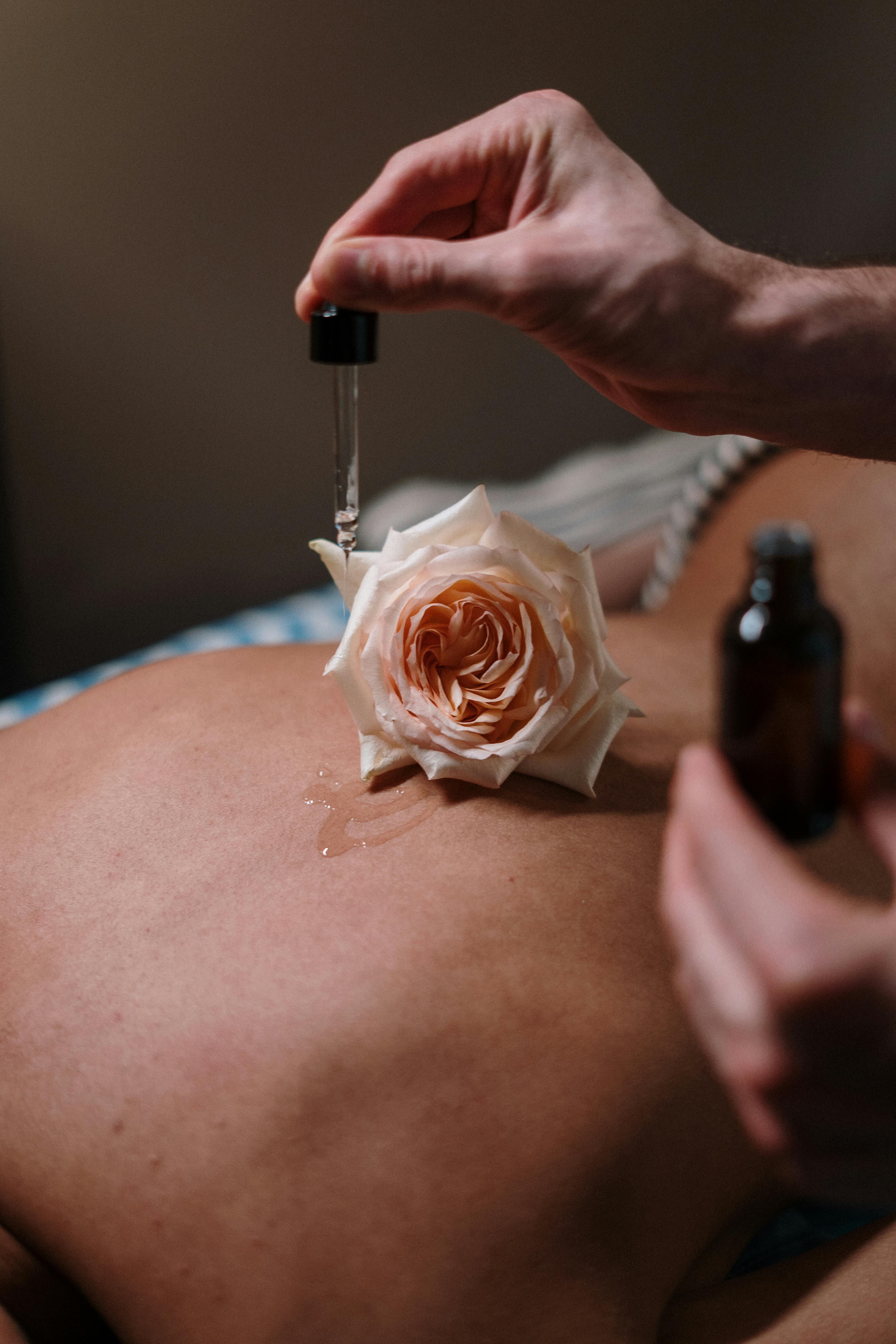
FAQs on Relative Frequency Calculation
1. What is the definition of relative frequency?
Relative frequency is defined as the ratio of the number of times a particular event occurs to the total number of observations. It reflects the likelihood of that event in the context of the total dataset, often expressed as a decimal, fraction, or percentage.
2. How do I calculate relative frequency from a frequency distribution?
To calculate relative frequency from a frequency distribution, divide each frequency count by the total number of observations within the dataset. Once calculated, you can express the relative frequency as a percentage for clearer interpretation.
3. Can relative frequency be used in qualitative data analysis?
Yes, relative frequency can be applied in qualitative data analysis to determine how often specific categories appear in the data. This helps in understanding the distribution of qualitative responses in surveys or studies.
4. Why is statistical significance important in frequency analysis?
Statistical significance is crucial as it determines whether the observed patterns in relative frequency are genuine or merely due to chance. Analyzing statistical significance ensures that conclusions drawn from data analysis are reliable and valid.
5. How can I improve my data collection techniques for better frequency results?
Improving data collection techniques encompasses employing systematic sampling methods, ensuring diverse participant representation, and utilizing validated survey tools. Continuous training on data collection best practices can also enhance data quality, facilitating accurate frequency measurement.